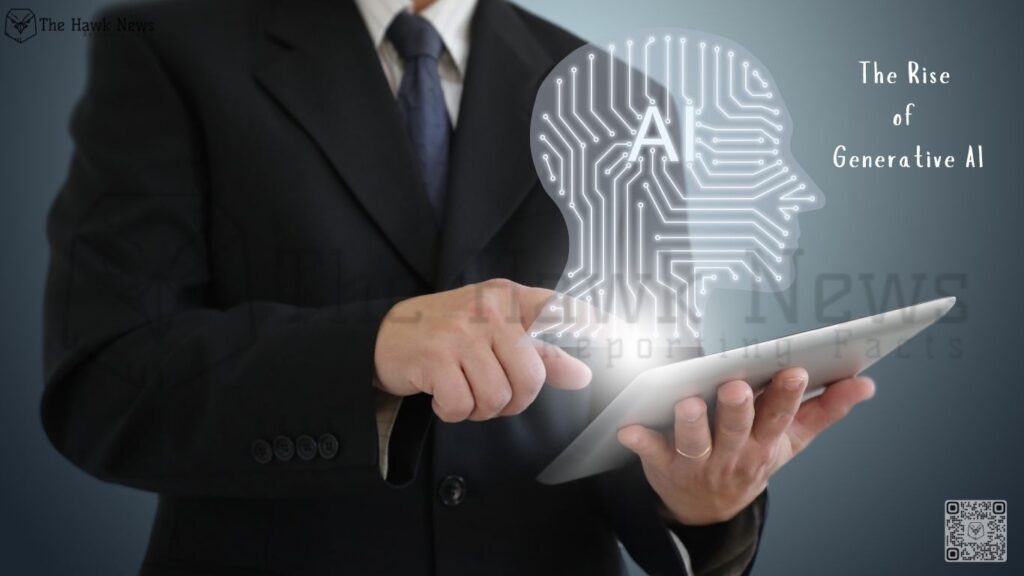
Artificial Intelligence (AI) has evolved from an abstract concept to a cornerstone of modern technology, reshaping industries and daily life. The journey of AI spans decades, filled with breakthroughs, challenges, and groundbreaking achievements. This article takes a deep dive into the key moments that have defined and advanced AI, highlighting the pivotal technologies, theories, and innovations that have propelled it from theory to the forefront of technological progress.
1. Alan Turing and the Concept of Machine Intelligence (1950)
The foundation of AI began with British mathematician Alan Turing, whose seminal 1950 paper, “Computing Machinery and Intelligence,” introduced the question: “Can machines think?” Turing proposed the Turing Test—a criterion to evaluate a machine’s ability to exhibit human-like intelligence. In this test, if a machine could converse with a human without being detected as a machine, it would be considered intelligent.
Turing’s work laid the philosophical and theoretical groundwork for AI, igniting debates about the nature of intelligence and the potential for machines to simulate it. The Turing Test remains a widely recognized benchmark in AI, serving as a conceptual starting point for the field.
2. The Dartmouth Conference: Birth of AI as a Field (1956)
The 1956 Dartmouth Conference is often regarded as the official birth of artificial intelligence as a scientific discipline. Organized by John McCarthy, Marvin Minsky, Nathaniel Rochester, and Claude Shannon, the conference brought together leading researchers to discuss the possibilities of creating intelligent machines. Here, the term “artificial intelligence” was coined, and the ambitious goal of creating machines that could perform tasks associated with human intelligence—such as problem-solving, learning, and reasoning—was set.
The Dartmouth Conference marked a turning point by establishing AI as a legitimate area of study and research, with a vision to mimic human cognitive functions. This early enthusiasm spurred initial funding and research, leading to subsequent discoveries and advancements.
3. The Rise of Expert Systems (1970s-1980s)
In the 1970s and 1980s, the focus of AI shifted toward expert systems, which were designed to mimic the decision-making abilities of a human expert in specific fields like medicine and engineering. These systems used rule-based approaches, relying on “if-then” rules to solve complex problems.
A prime example was the MYCIN system, developed at Stanford University, which could diagnose certain blood infections and recommend treatments based on its programmed medical knowledge. Expert systems demonstrated that AI could be applied practically in real-world scenarios, attracting investment and increasing AI’s influence across industries.
While expert systems highlighted the potential for AI in specialized applications, they also revealed limitations, as these systems struggled with tasks requiring flexibility, adaptability, or creativity. Nonetheless, expert systems played a key role in establishing AI’s utility, setting the stage for further innovation.
4. AI Winters and the Challenge of Progress (1970s and 1980s)
Despite initial successes, AI research encountered significant setbacks during periods known as AI winters. In the 1970s and late 1980s, funding and interest in AI waned due to unmet expectations and the limited capabilities of early AI systems. Challenges included insufficient computational power, limited data storage, and the high cost of developing complex systems.
These AI winters highlighted the gap between the ambitious goals set by researchers and the technological capabilities of the time. However, these challenges also spurred the development of new approaches and set the stage for later breakthroughs. Each AI winter ultimately helped refine goals, methods, and applications, building resilience within the AI research community.
5. The Emergence of Machine Learning (1980s-1990s)
During the late 1980s and 1990s, researchers began exploring machine learning as a new approach to AI. Unlike traditional AI systems, which rely on explicit programming and rule-based systems, machine learning emphasizes data-driven learning, where algorithms can learn from and improve through experience.
Machine learning algorithms, such as decision trees and neural networks, became popular as they allowed systems to handle more diverse and complex tasks. Notably, the backpropagation algorithm—a method for training neural networks—enabled more efficient learning and paved the way for future developments in deep learning.
This era saw the rise of supervised and unsupervised learning, which allowed systems to recognize patterns, make predictions, and classify data without pre-programmed instructions. Machine learning transformed AI, leading to applications in speech recognition, image analysis, and natural language processing.
6. IBM’s Deep Blue Defeats Chess Grandmaster Garry Kasparov (1997)
In 1997, IBM’s Deep Blue made history by defeating the world chess champion, Garry Kasparov. Deep Blue’s victory was a milestone that showcased AI’s potential to perform complex, strategic tasks traditionally associated with human intelligence. The computer’s ability to evaluate millions of possible moves per second and make decisions within seconds represented a significant leap in computational power and algorithmic design.
Deep Blue’s victory was not just a milestone in gaming; it symbolized the potential of AI to handle vast data and outperform humans in specific, rule-based tasks. This achievement inspired further research into developing systems that could handle other complex challenges, leading to breakthroughs in fields such as finance, logistics, and predictive analytics.
7. The Advent of Deep Learning and ImageNet (2012)
The 2010s marked a new era in AI with the advent of deep learning, a subset of machine learning based on artificial neural networks with many layers. Deep learning allowed for the automatic extraction of features from data, leading to significant advancements in tasks like image and speech recognition.
A pivotal moment was the ImageNet Large Scale Visual Recognition Challenge (ILSVRC) in 2012, where a deep learning model called AlexNet won the competition with an unprecedented accuracy rate in image classification. Developed by Alex Krizhevsky, Ilya Sutskever, and Geoffrey Hinton, AlexNet’s success demonstrated the power of deep learning, sparking a surge in research and applications in fields such as healthcare, self-driving cars, and virtual assistants.
The success of deep learning, fueled by advancements in GPU technology and access to large datasets, revolutionized AI by allowing it to tackle more nuanced and complex problems.
8. AlphaGo and the Mastery of Go (2016)
In 2016, Google DeepMind’s AlphaGo achieved a groundbreaking feat by defeating the world champion Go player Lee Sedol. Go is a highly complex board game with more possible moves than atoms in the universe, making it a monumental challenge for AI.
AlphaGo’s victory was achieved using a combination of reinforcement learning and deep neural networks. Unlike previous AI systems that relied on predefined rules, AlphaGo learned from playing millions of games and developing its strategies. The success of AlphaGo underscored the potential of AI in learning complex patterns autonomously and solving problems once thought too intricate for machines.
AlphaGo’s achievement inspired reinforcement learning developments and demonstrated AI’s potential to tackle complex decision-making tasks, from healthcare to climate modeling.
9. The Rise of Generative AI: GPT and Beyond (2018-Present)
In 2018, OpenAI introduced GPT (Generative Pretrained Transformer), an AI model that could generate human-like text based on a prompt. GPT-2, GPT-3, and later models like GPT-4 represented milestones in natural language processing (NLP), showing the ability to understand and generate coherent text across a wide range of topics.
These models have fueled advances in language translation, content creation, and chatbots, with applications across customer service, education, and creative industries. The release of ChatGPT and similar conversational agents brought AI to mainstream use, making it accessible and applicable to everyday tasks.
The rise of transformer-based models marked a new frontier for AI, showcasing unprecedented capabilities in generating text, images, and code. This era of generative AI promises to reshape fields such as healthcare, entertainment, and education.
Conclusion: A Rapidly Evolving Frontier
The milestones in AI’s history reveal a field marked by continuous evolution, where breakthroughs often build upon past discoveries to push the boundaries of what machines can achieve. From Turing’s foundational questions to the generative powers of modern AI models, each achievement has shaped the trajectory of AI, leading us closer to a future where intelligent systems are integral to daily life.
As AI technology advances, it is likely to transform even more sectors, enhancing problem-solving, creativity, and innovation across industries. The milestones of the past are not just achievements; they are steps in an ongoing journey, with future breakthroughs promising to redefine what AI—and humanity—can accomplish together.